DC9. AI for road safety monitoring and crash prediction from micro- to macro levels
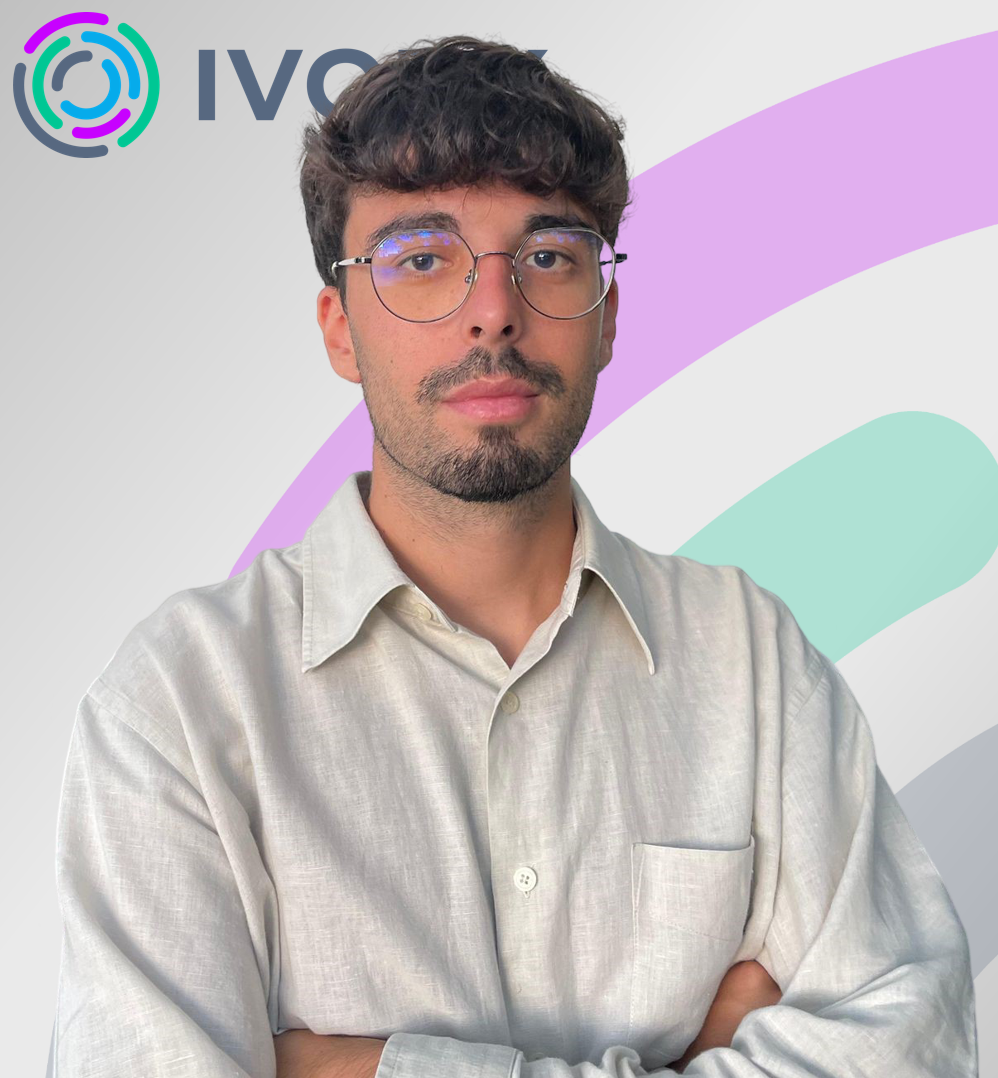
Simone Paradiso
Education
M.Eng in Management Engineering from University of Naples Federico II
About
Management engineer with strong sense of duty and logical aptitude for assigned tasks, carried out with great organizational and time management skills, even in difficult and pressured situations. The attitude towards analytics makes him enjoy gaining knowledge through the data exploration and analysis. Eager to learn and acquire new competencies and experience.
Hosts: NTUA & OSEVEN
Objectives:
- To investigate the effect of spatial scale on road safety monitoring and crash prediction
- To develop a new art AI framework to observe and analyse road safety KPIs and predict crashes by achieving transition from smaller scales (e.g., on a segment, intersection or neighbourhood level) to larger ones (e.g., highway corridor or prefecture/county level), taking into account the time dimension
- To assess the effectiveness and scalability of microscopic road safety models for macroscopic crash prediction and vice versa
Expected results:
- Evaluation of several scaling combinations that will also feature capabilities of ‘zooming in/zooming out’ of study areas using different levels of telematics (e.g., trip-based, driver-based or network-based using several drivers)
- Knowledge on comparable advantages and disadvantages for each analysis scale
- A case study utilising driver telematics in an urban area, with actionable results, compatible with the vision and activities of OSeven – showcasing the impact of using AI for micro-analysis based on driver telematics and integrating the findings to larger scales
Planned secondment(s):
- EIRA-SI, Purpose: to test the developed methods on additional datasets and countries, with an emphasis on transferability techniques