DC2. Explainable AI for road safety: benchmarking AI methods and data
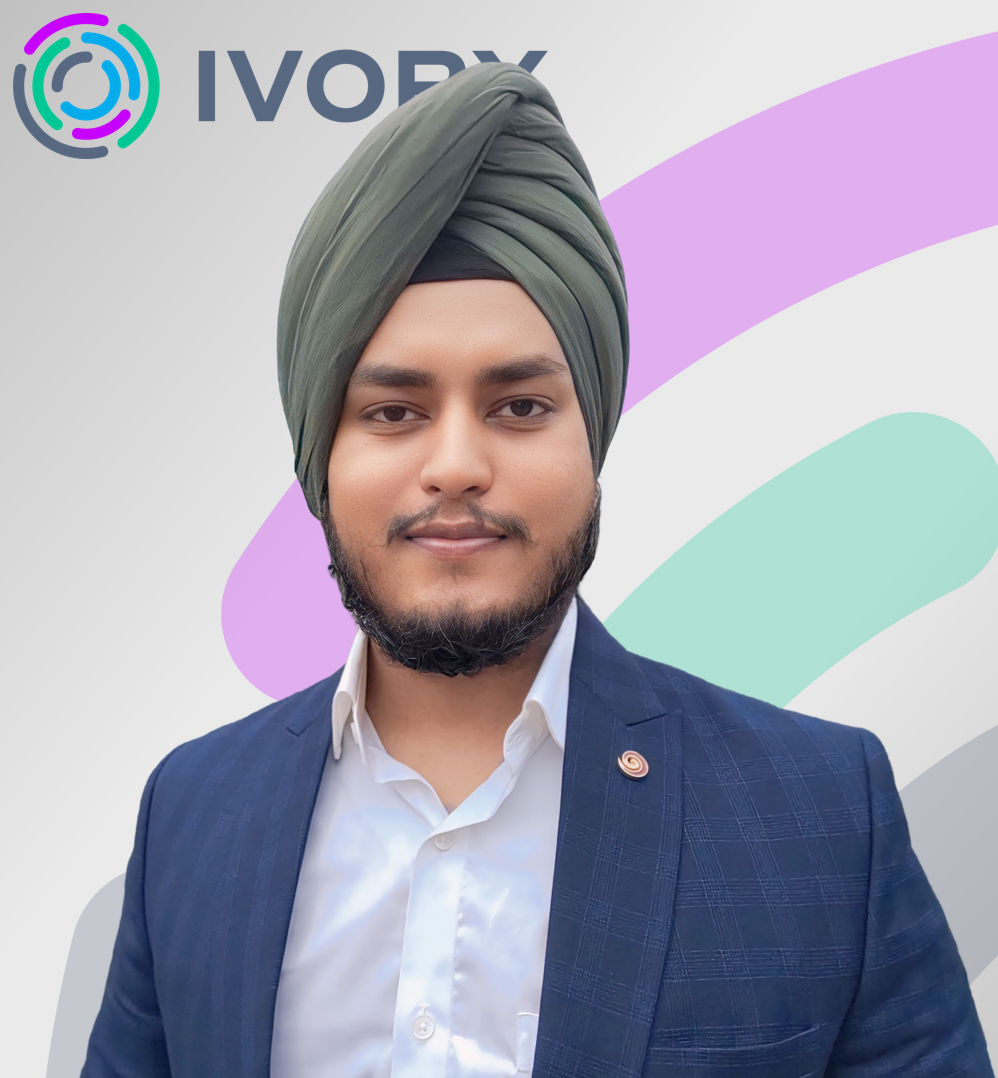
Manjinder Singh
Education
Mtech. in transportation engineering from IIT Delhi
About
With a strong foundation in civil engineering and a specialization in Transportation Engineering, Manjinder is a doctoral researcher interested in exploring the intersection of transportation economics, choice modelling, traffic safety and machine learning. He frequently works with the transportation research community and has published articles in peer-reviewed journals. His work in the Ivory project broadly involves analyzing the capabilities and limitations of statistical, econometric, and machine learning models in the context of road safety. Outside of academia, Manjinder enjoys exploring different cultures through travel and finds inspiration in various art forms, especially music.
Hosts: TUD & AGILYSIS
Objectives:
- To define the needs for explainability in AI for road safety, from the perspective of policy makers (transport authorities)
- To disentangle strengths and weaknesses (prediction accuracy and interpretability) of two types of AI methodologies –ML algorithms
- and statistical/econometric models
- To understand the performance and optimally integrate both techniques with benchmark datasets and applications in road safety for AI
- transparency in decision support
Expected results:
- A taxonomy of explainable AI methodologies and their applications in road safety
- A model-agnostic methodological framework for mixing ML algorithms and statistical/econometric methods
- A new explainable AI-based risk mapping tool for urban roads in West Midlands, UK
Planned secondment(s):
- Transport for West Midlands, Purpose: to incorporate the decision maker’s perspective on explainable AI and collect data for testing the explainable AI framework.