DC2. Explainable AI for road safety: benchmarking AI methods and data
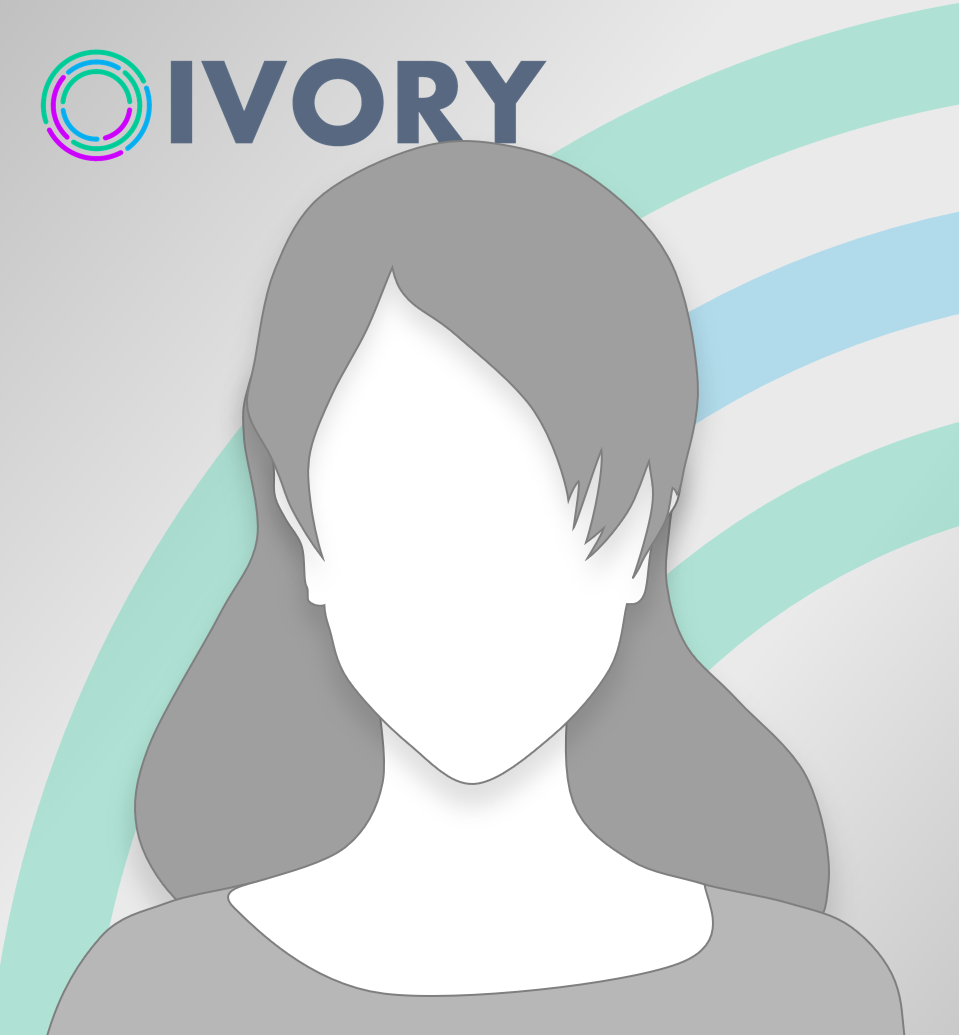
Hosts: TUD & AGILYSIS
Objectives:
- To define the needs for explainability in AI for road safety, from the perspective of policy makers (transport authorities)
- To disentangle strengths and weaknesses (prediction accuracy and interpretability) of two types of AI methodologies –ML algorithms
- and statistical/econometric models
- To understand the performance and optimally integrate both techniques with benchmark datasets and applications in road safety for AI
- transparency in decision support
Expected results:
- A taxonomy of explainable AI methodologies and their applications in road safety
- A model-agnostic methodological framework for mixing ML algorithms and statistical/econometric methods
- A new explainable AI-based risk mapping tool for urban roads in West Midlands, UK
Planned secondment(s):
- Transport for West Midlands, Purpose: to incorporate the decision maker’s perspective on explainable AI and collect data for testing the explainable AI framework.